The Need for AI in Document Processing
Traditional enterprises operate with multiple and often siloed business units. Paper-based workflows continue to dominate business unit processes, leading to challenges...
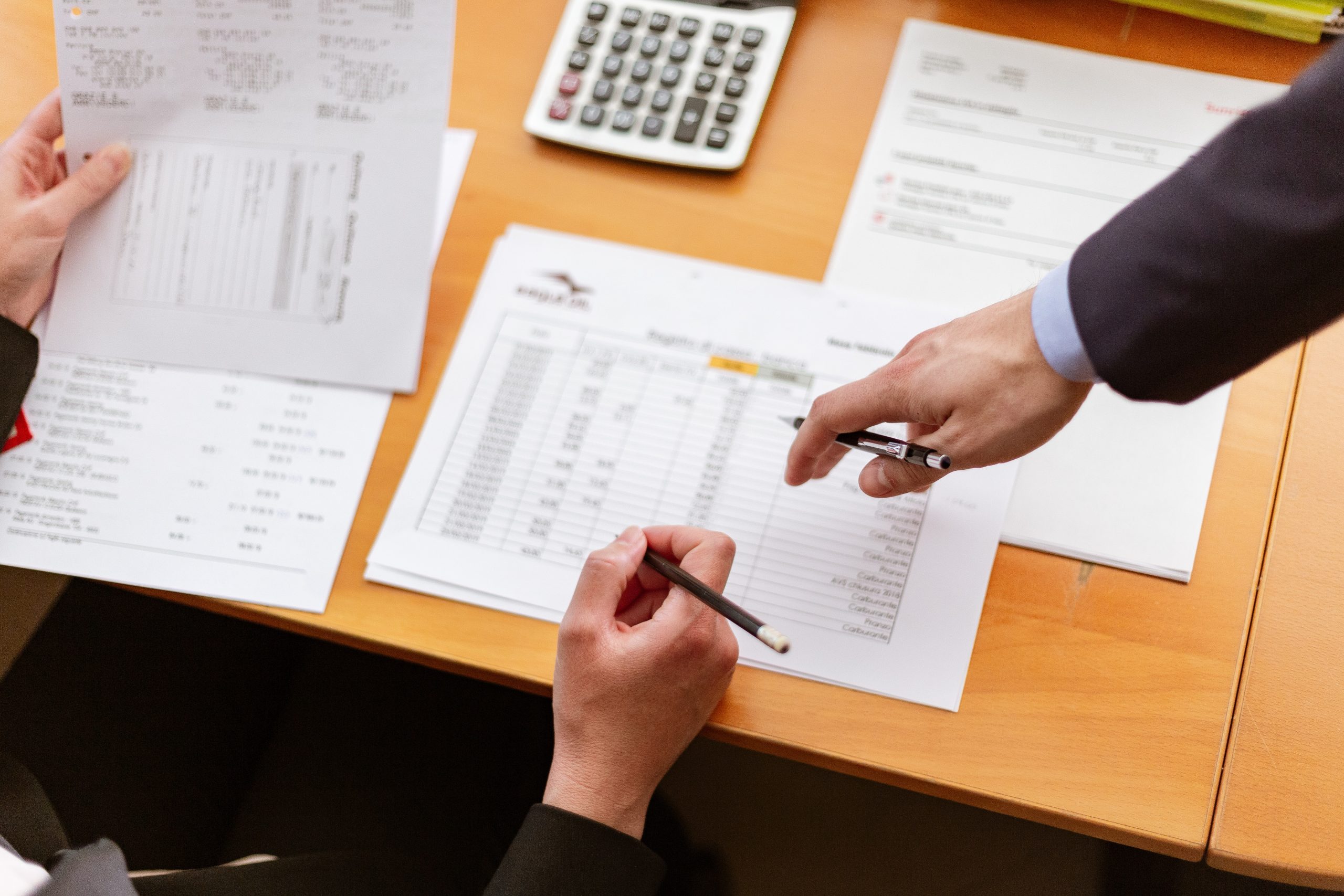
Traditional enterprises operate with multiple and often siloed business units. Paper-based workflows continue to dominate business unit processes, leading to challenges in extracting critical information. While documents are usually in digital formats (such as .PDF, .JPG, .PNG) the data within these docs must be carefully read and processed to extract relevant information. Most large Enterprises spend millions of dollars every year to extract this information manually which is time-consuming & error-prone and most importantly, isn’t scalable.
This is where Document AI comes into picture to extract the validated information and save data through appropriate workflows. In this fast-paced technology era, AI helps in addressing end-to-end automation for data extraction along with Optical Character Recognition (OCR) which minimizes the entire management process. Traditional OCR methodologies also sometimes fall short of accuracy benchmarks due to limited model training capabilities. Hence, deep learning frameworks have caught the attention of new-age business managers in order to streamline extensive document workflows that vary in templates.
How does it work?
1. Image Pre-processing
Image Pre-processing is a critical step and ML engineers spend most of the time cleansing the data before building the ML model. This step helps in enhancing the image quality by eliminating unwanted distortions which improves the data quality that benefits the ML model for further analysis.
2. Image Classification
Mapping the data from different domains has been quite challenging in document processing. Assigning a label to the image plays the most important role in image analysis. Deep learning techniques using Graph Neural Network (GNN) based classifiers give good accuracy in the output as they generalize the data as well as identify the invalid documents. GNN has now evolved into Graph Convolution Network (GCN) which is more efficient and forms the baseline for other complex GNN architectures.
3. Information Extraction
Once the data is classified, extracting relevant information is very crucial in the field of Natural Language Processing (NLP) and can be done in two ways – Traditional Information Extraction or Open Information Extraction. These techniques efficiently analyze unstructured data in the free form and give the output in structured form which is more valuable for data analysis.
Advantages
1. Highly Effective – Automation eliminates the manual intervention which leads to an increase in efficiency of the business work-flows sometimes even a whopping 75% decrease in operational expenses!
2. Processing time – Vast volumes of data can be processed way faster than it would take to process them manually.
3. Reliability – Tendency for error-prone workflows is quite minimal when it comes to repetitive tasks, unlike manual workflows.
4. Scalability – It is highly scalable and effective as it is a generalized process and can be applied to process documents across various domains.
Final thoughts
Considering the global pandemic situation, lots of businesses that generally deal with loads of paperwork are shifting to digital workflows and trying to reduce manual intervention. To enable a smooth transition of operational processes, AI-backed solutions come in handy in the industry and completely change the status quo while enabling competitive advantage.